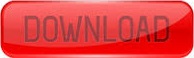
- #Series with time calc how to
- #Series with time calc software
- #Series with time calc code
- #Series with time calc series
#Series with time calc series
In this booklet, I will be using time series data sets that have been kindly madeĪvailable by Rob Hyndman in his Time Series Data Library at
#Series with time calc code
“Time series” (product code M249/02), available from Presented here, I would highly recommend the Open University book If you are new to time series analysis, and want to learn more about any of the concepts
#Series with time calc how to
To explain how to carry out these analyses using R. The principal focus of the booklet is not to explain time series analysis, but rather This booklet assumes that the reader has some basic knowledge of time series analysis, and That are common in analysing time series data.
#Series with time calc software
Now you are ready to move on to any dataset.This booklet itells you how to use the R statistical software to carry out some simple analyses Model.add(LSTM(256,input_shape = (trainx.shape, 2))) Model.add(LSTM(512, return_sequences = True, input_shape = (trainx.shape, 2))) You can run the code given below and play with the model parameters to see how the results change. Now, we should try and model a sine or cosine wave in a similar fashion. Now let’s see what our predictions look like. Model.fit(trainx, trainy, epochs = 2000, batch_size = 10, verbose = 2, shuffle = False) pile(loss = 'mean_squared_error', optimizer = 'adam') Model.add(LSTM(128,input_shape = (trainx.shape, 2))) Model.add(LSTM(256, return_sequences = True, input_shape = (trainx.shape, 2))) The epochs are to be run ‘til the time the error is reducing. Small batches of training data are shown to network, one run of when entire training data is shown to the model in batches and error is calculated is called an epoch. Testx = numpy.reshape(testx, (testx.shape, 1, 2)) Trainx = numpy.reshape(trainx, (trainx.shape, 1, 2)) Testx,testy = create_dataset(test, look_back) Trainx,trainy = create_dataset(train, look_back) Return numpy.array(dataX), numpy.array(dataY) When look-back period is 1, is converted to − Let’s convert the time series data into the form of supervised learning data according to the value of look-back period, which is essentially the number of lags which are seen to predict the value at time ‘t’. Now that the data has been created and split into train and test. Test = numpy.array(list(zip(trainx,trainy))) Train = numpy.array(list(zip(trainx,trainy))) Let us see, if LSTM can learn the relationship of a straight line and predict it.įirst let us create the dataset depicting a straight line. To understand the implementation of LSTM, we will start with a simple example − a straight line. Now that we have understood the internal working of LSTM model, let us implement it. Finally, the output gate decides which information should be passed on to the next hidden state The input gate controls the information flow to the current cell state using a point-wise multiplication operation of ‘sigmoid’ and ‘tanh’ respectively. The forget gate decides which information from the previous cell state should be forgotten for which it uses a sigmoid function.
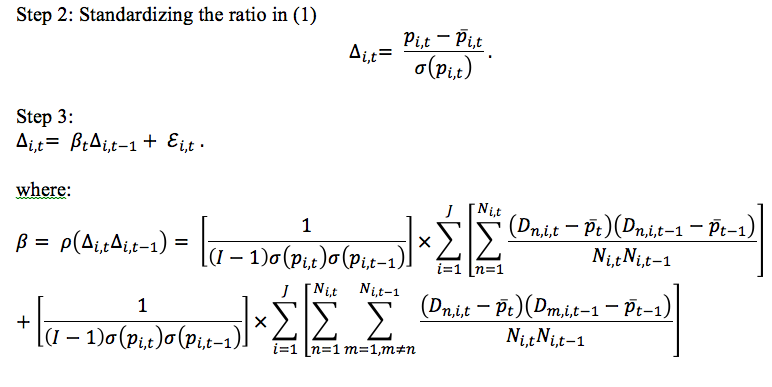
Each unit has an input, output and a forget gate which can add or remove the information to the cell state. The cell state in LSTM helps the information to flow through the units without being altered by allowing only a few linear interactions. An LSTM module has a cell state and three gates which provides them with the power to selectively learn, unlearn or retain information from each of the units. The picture above depicts four neural network layers in yellow boxes, point wise operators in green circles, input in yellow circles and cell state in blue circles. This is achieved because the recurring module of the model has a combination of four layers interacting with each other. It is special kind of recurrent neural network that is capable of learning long term dependencies in data.
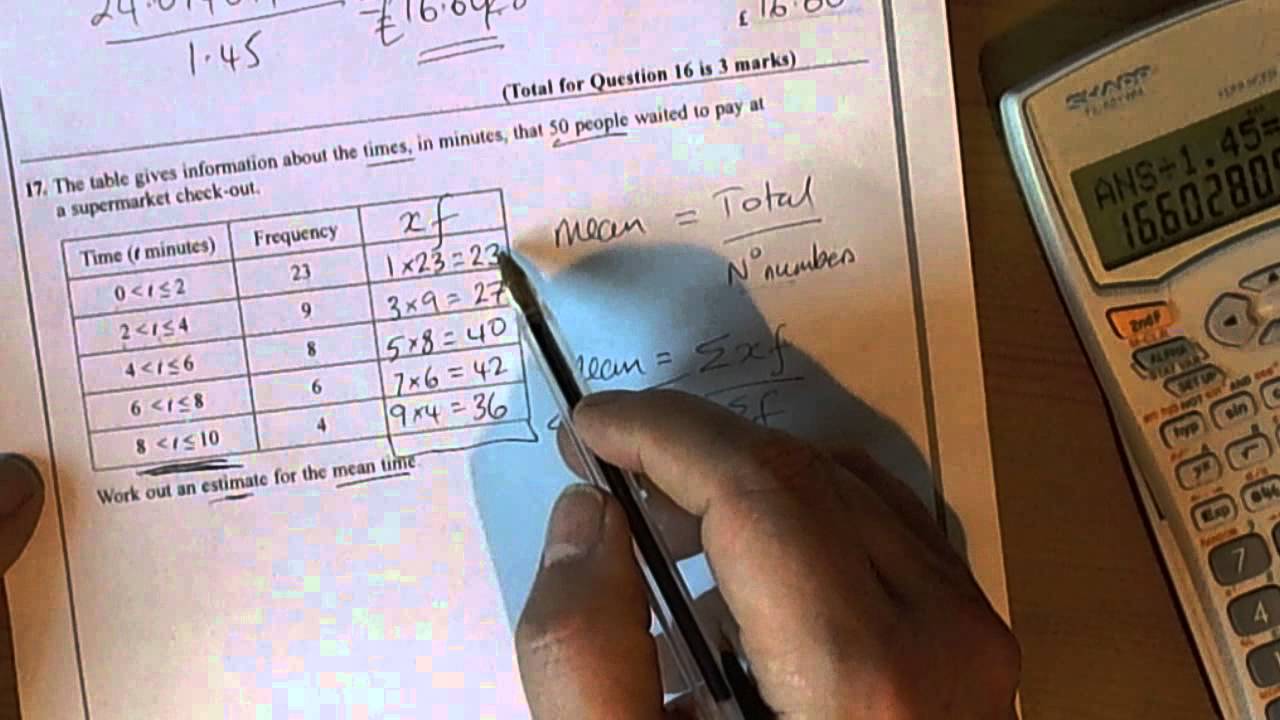
However, not for a long time, which is why we need LSTM models. There are recurring module(s) of ‘tanh’ layers in RNNs that allow them to retain information.
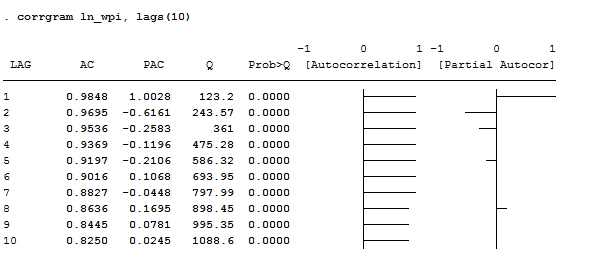
The neurons of RNN have a cell state/memory, and input is processed according to this internal state, which is achieved with the help of loops with in the neural network. It is a class of neural networks tailored to deal with temporal data. It is not one algorithm but combinations of various algorithms which allows us to do complex operations on data. Neural NetworksĪn artificial neural network is a layered structure of connected neurons, inspired by biological neural networks. So before we can jump to LSTM, it is essential to understand neural networks and recurrent neural networks. LSTM is a class of recurrent neural network. We shall start with the most popular model in time series domain − Long Short-term Memory model. Now, we are familiar with statistical modelling on time series, but machine learning is all the rage right now, so it is essential to be familiar with some machine learning models as well.
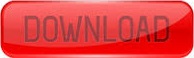